Detección automática de la Retinopatía Diabética ¿Mito o realidad?
Palabras clave:
Retinopatía, diabética, visión computacional, reconocimiento de patrones, inteligencia artificial
Resumen
Diabetic Retinopathy has become a worldwide health problem affecting diabetic people of working age, generating loss of vision or permanent blindness to patients, affecting their quality of life. Clinical detection methods are time consuming and expensive, which translates into a high incidence of this disease. Contemporary technological developments can be used to carry out the diagnosis of the disease, from the use of computer vision techniques that relate the areas of pattern recognition, image processing and artificial intelligence.
Descargas
La descarga de datos todavía no está disponible.
Citas
Budai, A., Bock, R., Maier, A., Hornegger, J., y Michelson, G. Robust Vessel Segmentation in Fundus Images. Int. J. Biomed. Imaging, 2013, pp. 1–11, 2013. https://doi.org/10.1155/2013/154860
Decencière, E., Zhang, X., Cazuguel, G., Laÿ, B., Cochener, B., Trone, C., Gain, P., Ordóñez-Varela, J. R., Massin, P., Erginay, A., Charton, B., y Klein, J. C. Feedback on a publicly distributed image database: The Messidor database. Image Anal. Stereol. https://doi.org/10.5566/ias.1155
Dutta, S., Manideep, B. C. S., Basha, S. M., Caytiles, R. D., y Iyengar, N. C. S. N. Classification of Diabetic Retinopathy Images by Using Deep Learning Models. Int. J. Grid Distrib. Comput., 11[1], pp. 99–106, 2018. https://doi.org/10.14257/ijgdc.2018.11.1.09
International Diabetes Federation. International Diabetes Federations response to the 3rd UN HLM on NCDs political declaration.
Martínez-Murcia, F. J., Ortiz, A., Ramírez, J., Górriz, J. M., y Cruz, R. Deep residual transfer learning for automatic diagnosis and grading of diabetic retinopathy. Neurocomputing, 452, pp. 424– 434, 2021. https://doi.org/10.1016/j.neucom.2020.04.148
Mateen, M., Wen, J., Hassan, M., Nasrullah, N., Sun, S., y Hayat, S. Automatic Detection of Diabetic Retinopathy: A Review on Datasets, Methods and Evaluation Metrics. IEEE Access, 8, pp. 48784– 48811, 2020. https://doi.org/10.1109/ ACCESS.2020.2980055
Porwal, P., Pachade, S., Kamble, R., Kokare, M., Deshmukh, G., Sahasrabuddhe, V., y Meriaudeau, F. Indian Diabetic Retinopathy Image Dataset (IDRiD). IEEE Dataport. https://doi.org/10.21227/H25W98
Porwal, P., Pachade, S., Kokare, M., Deshmukh, G., Son, J., Bae, W., Liu, L., Wang, J., Liu, X., Gao, L., Wu, T., Xiao, J., Wang, F., Yin, B., Wang, Y., Danala, G., He, L., Choi, Y. H., Lee, Y. C., … Mériaudeau, F. IDRiD: Diabetic Retinopathy – Segmentation and Grading Challenge. Med. Image Anal., 59, pp. 101561, 2020. https://doi.org/10.1016/j.media.2019.101561
Rio Torres, M., Fernández Argones, L., Hernández Silva, J. R., y Ramos López, M. Oftalmología: Diagnóstico y tratamiento (2a ed.). Ciencias Médicas.
World Health Organization. WHO guideline: recommendations on digital interventions for health system strengthening. World Health Organization
Decencière, E., Zhang, X., Cazuguel, G., Laÿ, B., Cochener, B., Trone, C., Gain, P., Ordóñez-Varela, J. R., Massin, P., Erginay, A., Charton, B., y Klein, J. C. Feedback on a publicly distributed image database: The Messidor database. Image Anal. Stereol. https://doi.org/10.5566/ias.1155
Dutta, S., Manideep, B. C. S., Basha, S. M., Caytiles, R. D., y Iyengar, N. C. S. N. Classification of Diabetic Retinopathy Images by Using Deep Learning Models. Int. J. Grid Distrib. Comput., 11[1], pp. 99–106, 2018. https://doi.org/10.14257/ijgdc.2018.11.1.09
International Diabetes Federation. International Diabetes Federations response to the 3rd UN HLM on NCDs political declaration.
Martínez-Murcia, F. J., Ortiz, A., Ramírez, J., Górriz, J. M., y Cruz, R. Deep residual transfer learning for automatic diagnosis and grading of diabetic retinopathy. Neurocomputing, 452, pp. 424– 434, 2021. https://doi.org/10.1016/j.neucom.2020.04.148
Mateen, M., Wen, J., Hassan, M., Nasrullah, N., Sun, S., y Hayat, S. Automatic Detection of Diabetic Retinopathy: A Review on Datasets, Methods and Evaluation Metrics. IEEE Access, 8, pp. 48784– 48811, 2020. https://doi.org/10.1109/ ACCESS.2020.2980055
Porwal, P., Pachade, S., Kamble, R., Kokare, M., Deshmukh, G., Sahasrabuddhe, V., y Meriaudeau, F. Indian Diabetic Retinopathy Image Dataset (IDRiD). IEEE Dataport. https://doi.org/10.21227/H25W98
Porwal, P., Pachade, S., Kokare, M., Deshmukh, G., Son, J., Bae, W., Liu, L., Wang, J., Liu, X., Gao, L., Wu, T., Xiao, J., Wang, F., Yin, B., Wang, Y., Danala, G., He, L., Choi, Y. H., Lee, Y. C., … Mériaudeau, F. IDRiD: Diabetic Retinopathy – Segmentation and Grading Challenge. Med. Image Anal., 59, pp. 101561, 2020. https://doi.org/10.1016/j.media.2019.101561
Rio Torres, M., Fernández Argones, L., Hernández Silva, J. R., y Ramos López, M. Oftalmología: Diagnóstico y tratamiento (2a ed.). Ciencias Médicas.
World Health Organization. WHO guideline: recommendations on digital interventions for health system strengthening. World Health Organization
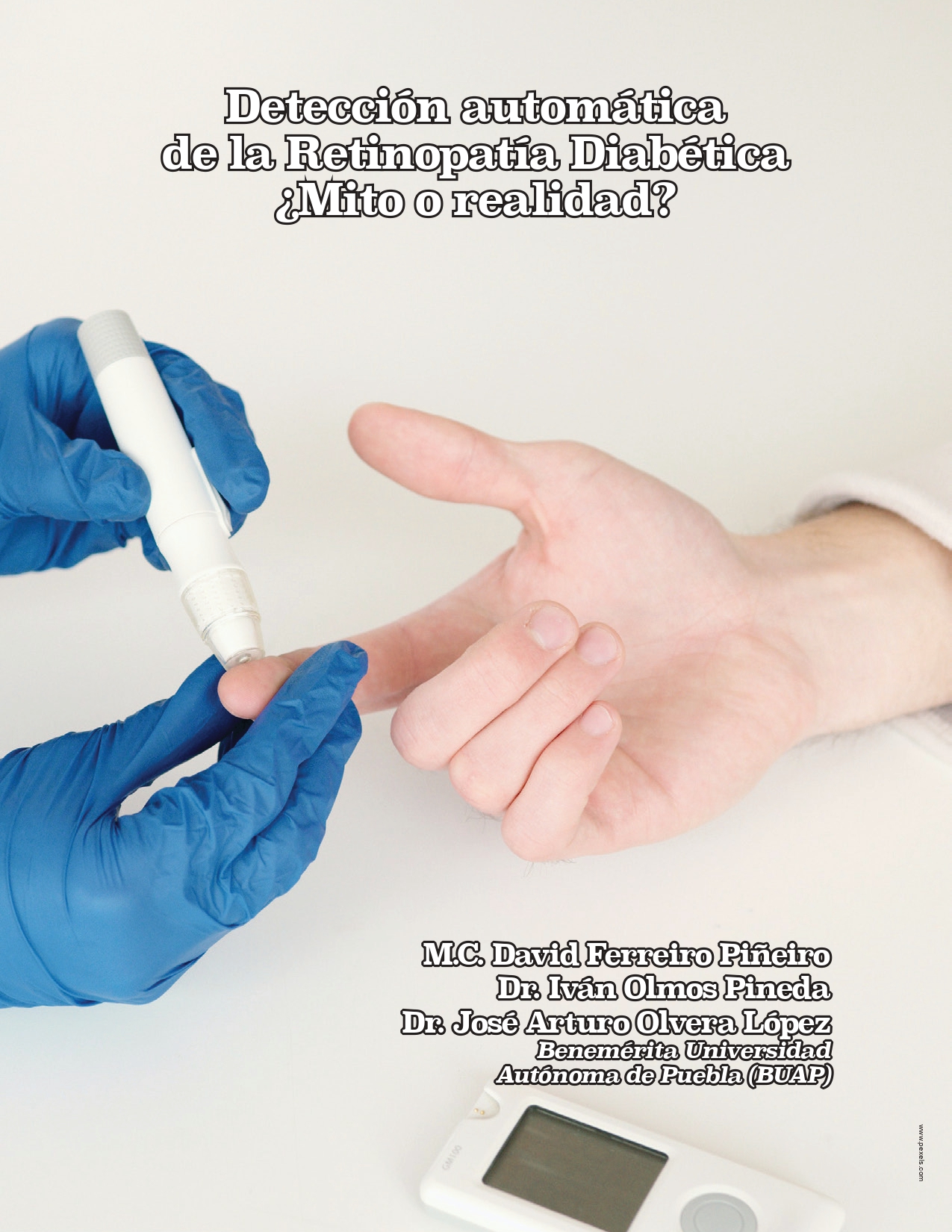
Publicado
2022-10-03
Cómo citar
Ferreiro-Piñeiro, D., Olmos-Pineda, I., & Olvera-López , J. A. (2022). Detección automática de la Retinopatía Diabética ¿Mito o realidad?. Contactos, Revista De Educación En Ciencias E Ingeniería, 1(125), 5-12. Recuperado a partir de https://contactos.izt.uam.mx/index.php/contactos/article/view/218
Sección
Artículos